Unlocking the Potential of Image Annotation in Machine Learning
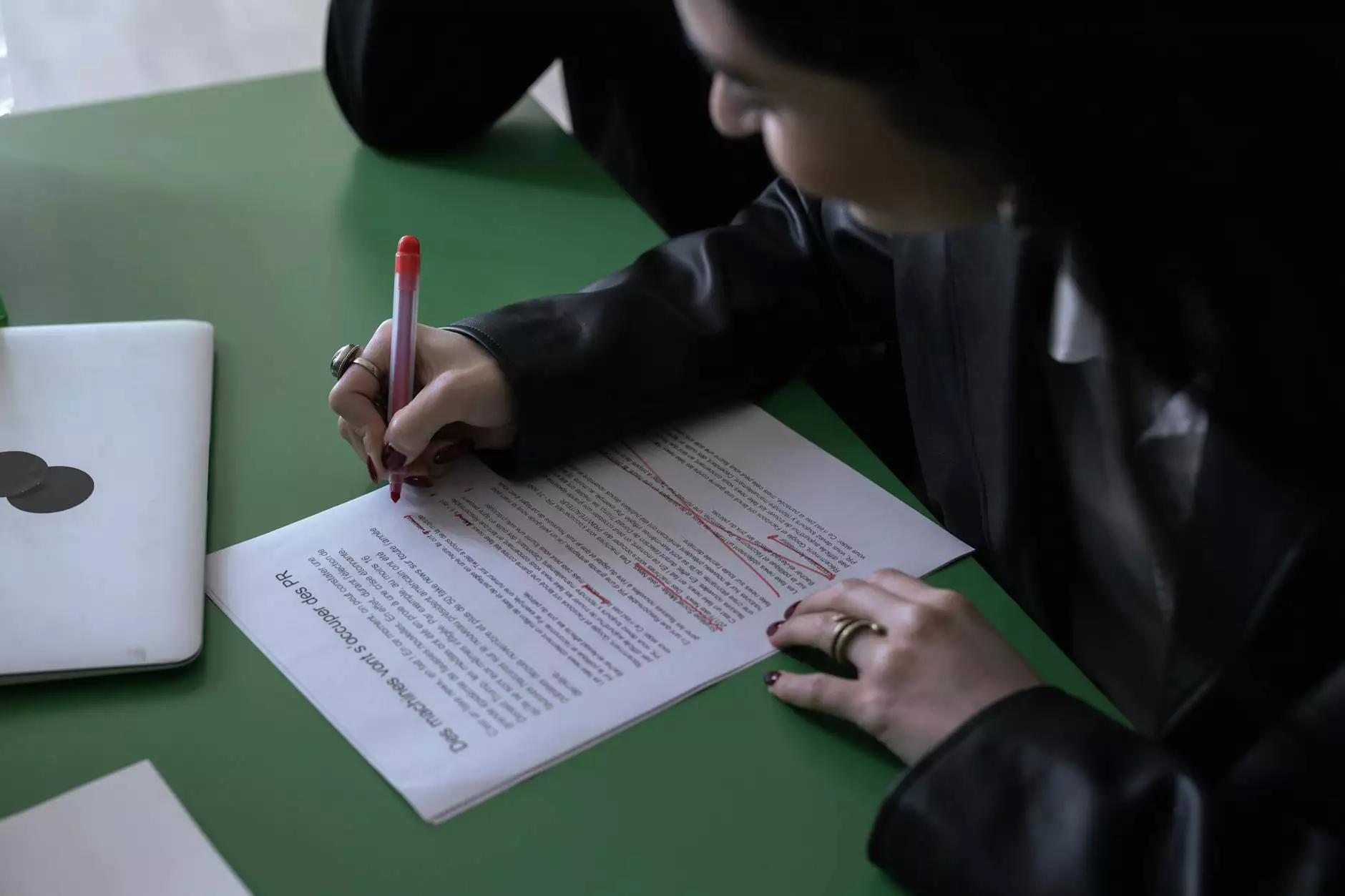
What is Image Annotation?
Image annotation is the process of labeling images to provide context that machine learning algorithms need to learn. In the digital age, visual data is immensely valuable, as it feeds into various applications ranging from automated vehicle systems to sophisticated healthcare diagnostics. The critical function of image annotation is to ensure that computers can identify and understand objects within images, thus enhancing their decision-making capabilities.
Importance of Image Annotation in Machine Learning
In the realm of machine learning, particularly in computer vision, the quality and accuracy of training data significantly influence model performance. Here are several reasons why image annotation plays a vital role:
- Enhanced Model Accuracy: Properly annotated images provide clear examples of the target objects, which helps machine learning models learn effectively.
- Facilitating Supervised Learning: Supervised learning relies on labeled data for training. Image annotation is crucial for creating these datasets.
- Improving Object Detection Algorithms: Annotated datasets help algorithms in identifying and categorizing various objects in images with high precision.
Types of Image Annotation Techniques
Image annotation encompasses a variety of techniques, each aimed at serving different needs of machine learning applications:
1. Bounding Box Annotation
This is one of the most commonly used methods. It involves drawing a rectangle around an object in an image to identify it. This technique is particularly useful in real-time object detection.
2. Polygon Annotation
When objects require a more precise outline, polygon annotation is preferred. It involves marking vertices that create a polygon around complex shapes, which is crucial in applications like autonomous driving.
3. Semantic Segmentation
This technique involves classifying each pixel in an image. It's an advanced form of image annotation that helps in understanding the underlying structures in images, particularly in fields like medical imaging.
4. Keypoint Annotation
In this method, specific points, or landmarks, are identified on an object. This is particularly useful in analyzing poses or tracking movements in video sequences.
Why Choose Keylabs for Your Image Annotation Needs?
When it comes to data annotation, Keylabs.ai stands out as a premier choice. Here’s why:
- Cutting-Edge Technology: Keylabs utilizes advanced AI technologies to assist in the annotation process, ensuring high accuracy and efficiency.
- Scalable Solutions: Our data annotation platform can handle projects of all sizes, catering to both small startups and large enterprises.
- Expert Team: With a team of experienced annotators, Keylabs guarantees meticulous attention to detail in every annotation task undertaken.
- Quick Turnaround Time: We respect deadlines and strive to deliver high-quality annotations in a timely manner.
The Role of Data Annotation Tools in Machine Learning
Data annotation tools are crucial for streamlining the image annotation process. These tools enhance productivity and ensure consistent quality across large datasets. Some notable features of effective data annotation tools include:
- User-Friendly Interface: A clean and intuitive interface allows annotators to work efficiently without a steep learning curve.
- Multiple Annotation Formats: The ability to support various annotation styles (bounding boxes, polygons, etc.) caters to diverse project needs.
- Collaboration Features: Real-time collaboration allows teams to work together seamlessly, improving efficiency and output quality.
Challenges in Image Annotation
While image annotation serves as a backbone for machine learning systems, it is not without its challenges:
1. Data Quality and Consistency
Ensuring high data quality and consistent annotations can be difficult, especially when large datasets are involved. Discrepancies can lead to decreased model performance.
2. Time-Consuming Process
Manual annotation can be a labor-intensive task, requiring significant resources and time to accurately label large volumes of images.
3. Need for Expert Knowledge
Some projects necessitate domain-specific knowledge to label data effectively, underscoring the need for skilled annotators.
Future Trends in Image Annotation and Machine Learning
The domain of image annotation is ever-evolving, and several trends are likely to shape its future:
- Automation of Annotation Processes: Machine learning models will play a bigger role in automating the annotation process, reducing human effort substantially.
- Use of Synthetic Data: The incorporation of synthetic data for training models will help supplement real-world annotated datasets, particularly in scenarios where data is scarce.
- Enhanced Augmented Reality Applications: As AR technology advances, image annotation techniques will increasingly be applied in real-time environments.
Conclusion
In conclusion, image annotation is an indispensable component of the machine learning landscape. Its ability to enhance model accuracy and facilitate the learning process cannot be overstated. At Keylabs.ai, we understand the complexities and challenges of data annotation and offer cutting-edge solutions tailored to meet the specific needs of our clients. Utilizing advanced technology and a skilled team, we aim to deliver the highest quality annotations that empower your machine learning projects. Harness the power of image annotation today and drive your AI initiatives forward!
image annotation machine learning